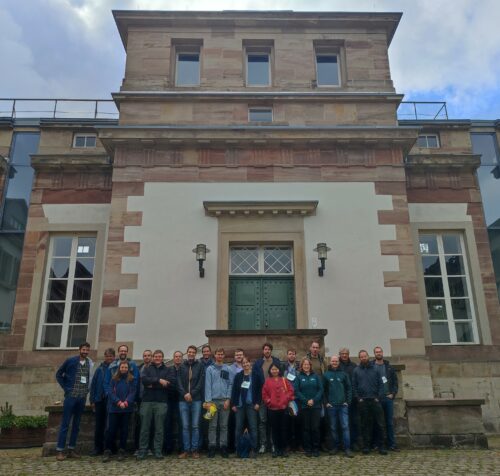
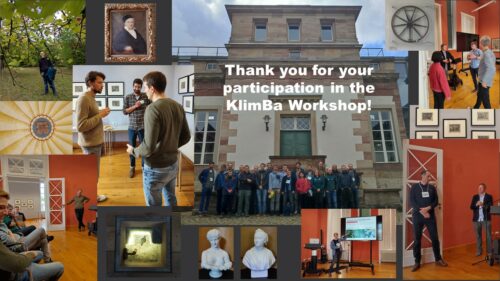
Our mid-term Workshop took place in September in Göttingen. We had the luck to spend 2 days in a historical location – The University Observatory – which served as the place of residence and work of famous scholar Carl Friedrich Gauss (1777–1855).
We could listen to interesting presentations of scientists studying the forest by remote sensing: David Frantz from Trier University, Jonathan Költzow from Future Forest Projekt, Hanna Meyer from WWU Münster and Maciej Lisiewicz from Forest Research Institute in Poland. Thank you for your presentations!
Take home message
- The German NFI was not designed to collect reference data
- The provision of research data is a byproduct of the NFI, not the original mandate
- The NFI is, however, interested in supporting research
- The general approach for generating reference data from single tree detection and linking to field inventory data will be made available so that it can be reproduced by other organizations (NFIs, management inventories)
- Forest management inventories are another, potentially valuable, data source
- Covariates, such as terrain features, site conditions, etc., are important in addition to the spectral signatures from the satellites and will be included in the final reference data base
- Applying the single tree approach allows for compiling a relatively universal reference data set
- Information on disturbance (biotic, abiotic) are important and a flag, based on available disturbance maps, will be added, up to which time an observation is valid
- Since the reference data base extracts spectral signatures as observed by the Sentinel-2 satellites, processed using the FORCE engine, this data will also be made available
- Linking field measured trees to ITD segments remains challenging. Advanced pattern matching algorithms will be tested
- Measuring the exact plot positions with differential GNSS receivers should become a standard in forest inventories
- The NFI data from 2022 will enlarge the data base significantly
- Interest in all levels of project output:
- Reference data base
- Deep Learning models for tree segmentation using high resolution (about 20 cm) RGB imagery
- Deep Learning models for tree species classification
- Tree species maps
Dear Participants of the Workshop – Thank you for your participation and lively discussions!
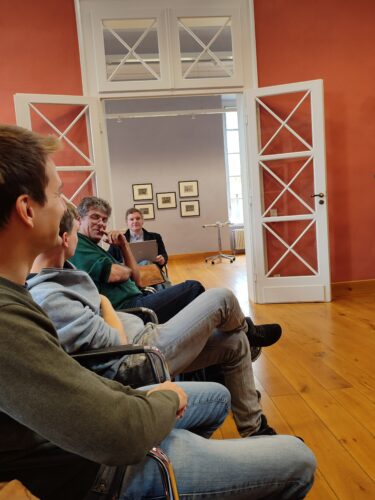
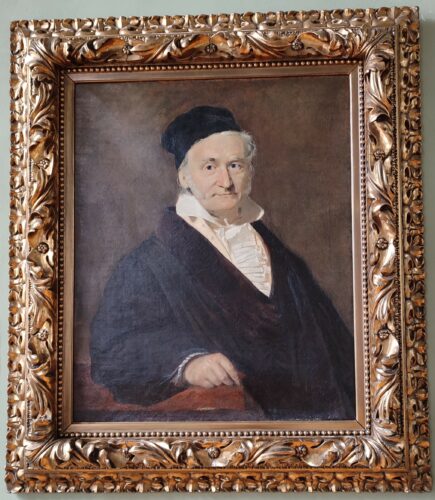
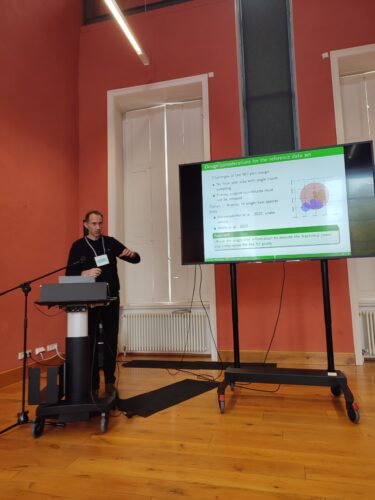
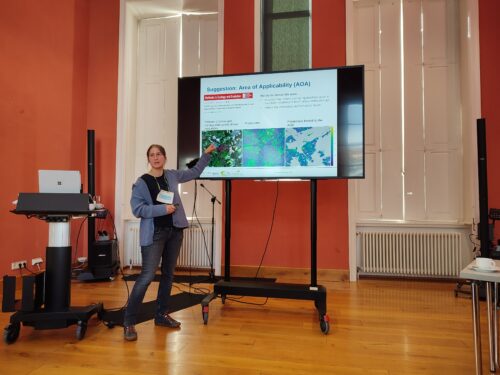
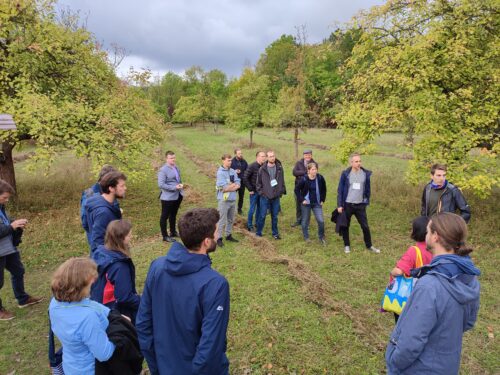
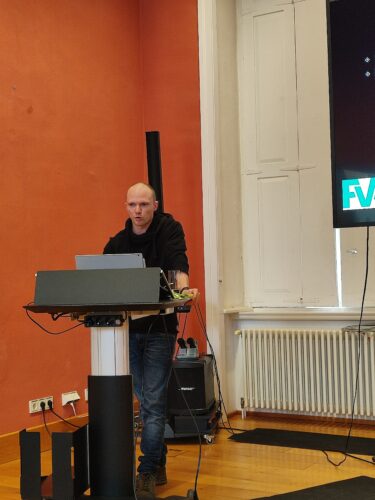
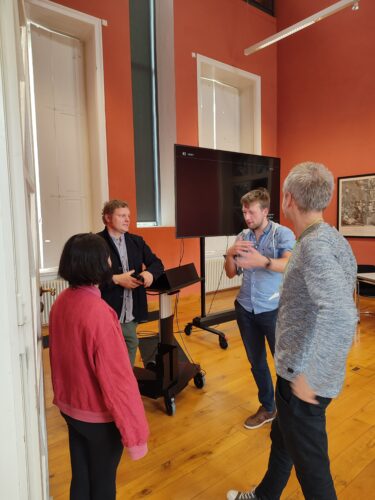
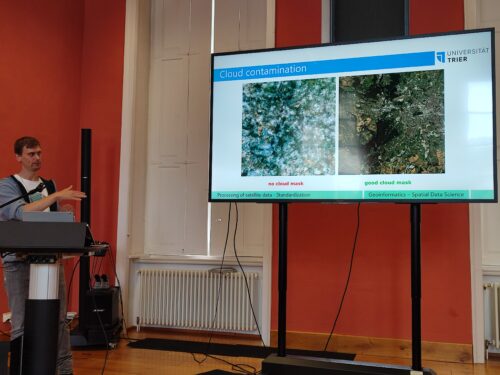
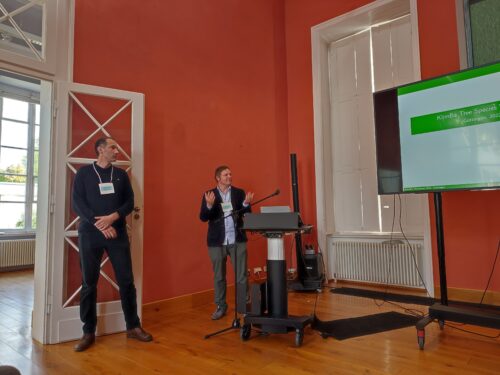
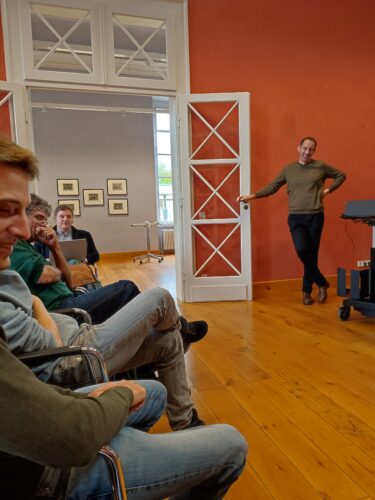
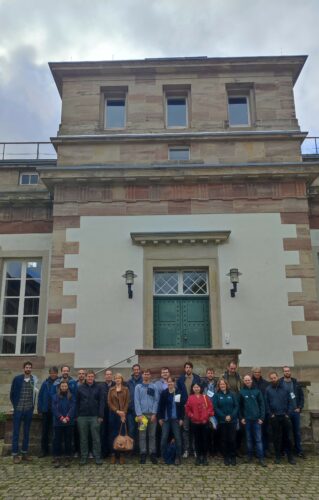
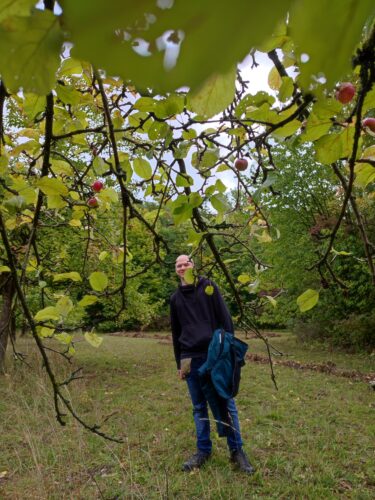